Cybersecurity threats never sleep. They only evolve. The latest twist? Artificial intelligence tools in the wrong hands. These powerful technologies have changed how we work. They’ve also opened new doors for criminals and hackers.
Bad guys now use AI to create more believable scams. They build attacks that learn and adapt. They find weak spots in your security faster than ever. This isn’t science fiction. It’s happening right now.
Last month, my client almost lost $200,000. Their CFO received a phone call from the “CEO” requesting an urgent wire transfer. The voice matched perfectly, and the number looked right. Only a random verification question stopped the fraud. The culprit? AI-generated voice cloning.
This article breaks down the real AI threats facing your business today. I’ll show you what these attacks look like. I’ll give you practical steps to protect yourself. The risks are serious, but you can fight back.
AI-Created Security Threats
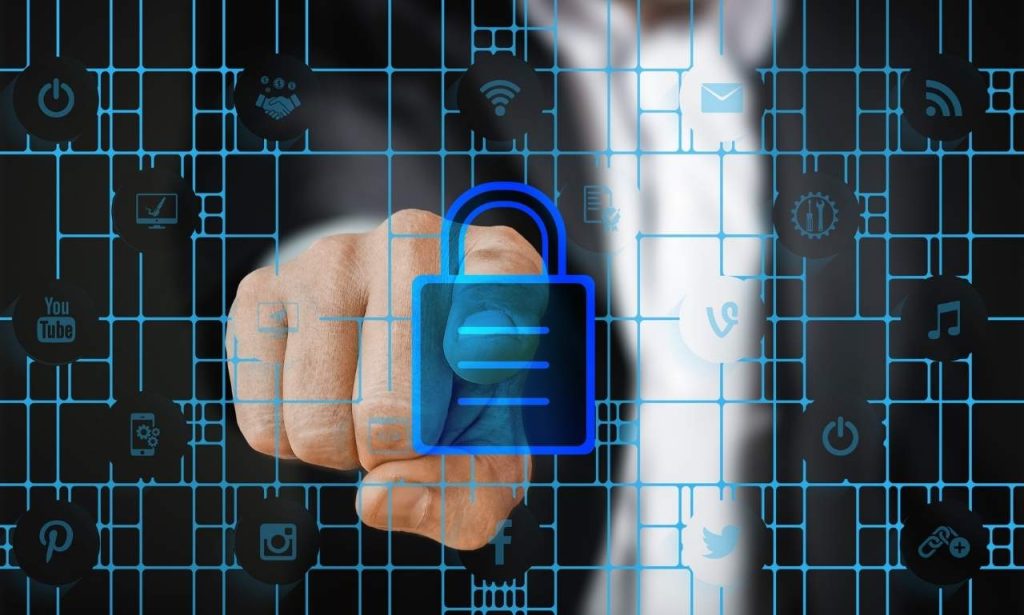
AI has changed the game for cybercriminals. The tech makes their attacks smarter and harder to spot. It lets them hit more targets with less work. The scary part? These tools keep getting better and cheaper.
A security chief at a mid-sized bank told me something telling. His team saw AI-assisted attacks jump 180% in just six months. Their old security tools missed many of these threats. The patterns looked different. The attacks adapted quickly. The scale was massive.
Companies face stolen data, financial loss, and damaged reputations. Regular people risk identity theft and empty bank accounts. Let’s look at exactly how these attacks work.
AI-Powered Social Engineering Attacks
Social engineering tricks people through psychological manipulation. AI makes these tricks far more convincing. Language models write perfect phishing emails with no spelling mistakes. They copy writing styles of people you trust. They create urgent messages that make you act before thinking.
Voice cloning might be the scariest new tool. Last week, a manufacturing executive I work with received a call from a voice that sounded exactly like his production manager. The call requested emergency access to certain systems and seemed to come from the manager’s number. Only a special code word system prevented disaster.
These attacks succeed because they feel personal. AI analyzes your social media to learn personal details, figure out your work relationships, and know when you typically check your messages. The fake communications arrive at your most vulnerable moments.
What’s worse, these tools get easier to use every month. What once required coding skills now has simple interfaces. Anyone can create fake messages that look and sound real. The old rules no longer apply.
Deep Fakes and Identity Fraud
Deepfake technology creates incredibly realistic fake videos and audio. The quality improves every few months, and sometimes, even experts can’t tell what’s real anymore.
A retail chain in Michigan learned this lesson the hard way. Staff watched what looked like their regional director announcing urgent protocol changes. The deepfake video almost caused massive data exposure. A quick phone call revealed the director knew nothing about it.
Deep fakes hurt both companies and regular people. They make us question real videos and recordings, enable new types of blackmail, and damage reputations with fake evidence. And the technology just keeps improving.
Adversarial AI Attacks
Adversarial attacks target the AI systems themselves. These clever attacks change inputs slightly to confuse AI tools. The changes often look normal to humans but completely fool the machines.
Self-driving car research shows the dangers. Tests proved that small stickers on road signs confused the AI. A stop sign with certain marks registered as a yield sign, and the car drove through without stopping. Similar tricks could target facial recognition, medical AI, or banking systems.
These attacks still require technical know-how, but that barrier keeps getting lower. Research papers explain the techniques, and online tools make them easier to use. As AI spreads to more systems, the risks multiply.
Evasion and Prompt Injection Attacks
Evasion attacks help bad actors slip past AI security tools. Small changes to malware code confuse detection systems. The tweaks mean nothing to humans but completely trick the machines into seeing safe code.
Prompt injection attacks represent a newer threat. This technique manipulates AI chatbots through carefully worded questions. It can make language models reveal private information. Consequently,iIt might bypass content filters. It could enable access to systems you want to protect.
A healthcare company discovered this problem recently. Their patient support chatbot started giving out sensitive information after specific questions. The attack bypassed several security measures. It exploited how the AI system was designed. The fix cost hundreds of thousands of dollars.
AI Model Poisoning and Backdoors
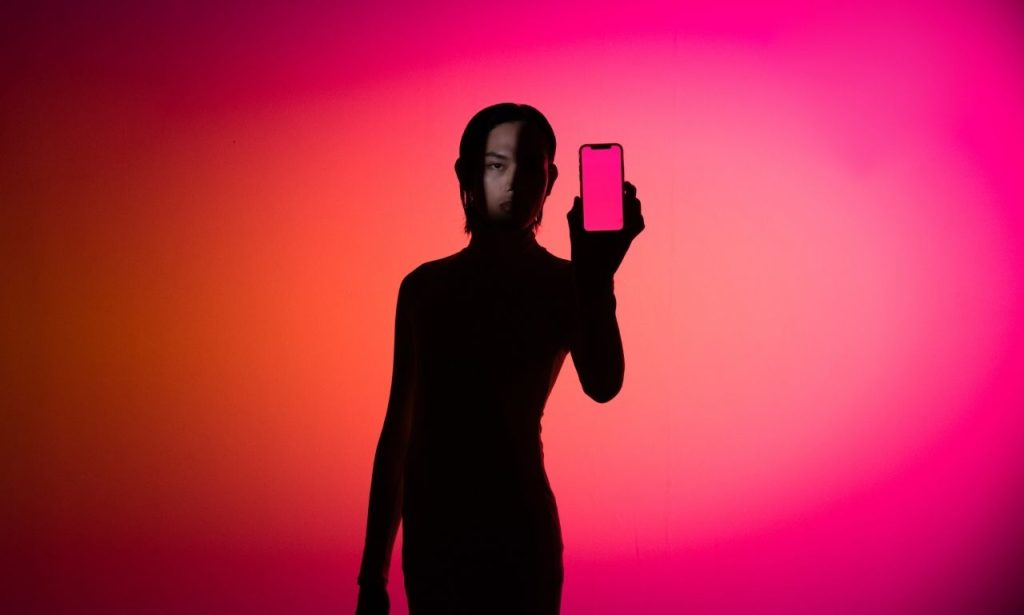
AI needs training data. The vulnerability exists because of poisoning attacks, which create a point of entry. The attackers embed destructive data samples into the training datasets. AI absorbs harmful behaviors during training that activate at later stages. The damage remains concealed until it gets activated.
The method proves highly effective for injecting poison into publicly available datasets. Several businesses operate using data that exists without cost. Checking every training example during development occurs infrequently. Bad actors use poisoned data to spread throughout training datasets. The harmful effects of model poisoning spread throughout the AI networks, such as viruses infecting systems.
The results from AI systems transform according to their operational activities. The implementation of poison into a shopping system could result in the promotion of particular products. When security tools become compromised, they fail to detect specific attacks. Content filtering systems with contamination issues will permit dangerous content to pass through. AI adoption expansion leads to an increased frequency of these risks.
Backdoor Triggers and Model Theft
Backdoor attacks work like time bombs in AI. Attackers train models to act normal except when triggered. A specific phrase or image activates the bad behavior. The model seems fine during testing, but the backdoor only shows up under specific conditions.
Model theft poses another big threat. Valuable AI systems represent serious intellectual property. Competitors or foreign governments might steal these assets. They could extract model details through careful questioning. They might use this knowledge to copy systems or find weaknesses.
A financial AI company experienced this firsthand. Their loan approval model showed signs of extraction. Someone had systematically tested the system with specific inputs. The pattern suggested a deliberate attempt to copy the model. The company lost years of competitive advantage.
Data Manipulation and Data Poisoning
AI completely depends on its data, which creates a critical weak spot through data manipulation. Attackers can poison training data or mess with input data, and the effects spread through all AI-powered decisions.
A retail analytics company learned this lesson painfully. Their customer prediction system suddenly made strange recommendations. An investigation showed that someone had tampered with historical sales data. Marketing decisions based on bad data cost millions in wasted campaigns.
Data poisoning typically targets systems during development, but running systems face risks, too. Live data feeds might include harmful inputs, and feedback systems could slowly corrupt AI behaviors. The damage gets harder to spot over time.
Targeting Feedback Systems
Many AI systems learn continuously from user feedback. This creates openings for manipulation. Coordinated campaigns can influence recommendation systems. They can skew content moderation decisions. They can trick market prediction algorithms.
A social media platform noticed this problem last quarter. Their content system showed unusual patterns. Investigation revealed organized efforts to promote specific viewpoints. Users with certain behaviors received increasingly extreme content. The attack exploited how the feedback system worked.
These attacks damage individual systems and broader trust. They potentially shape public opinion, might affect buying decisions, or might affect political views. They represent a growing threat to information systems everywhere.
AI-Enabled Malware and Ransomware
Traditional malware follows predictable patterns, making detection possible. AI-powered malware changes everything. It adapts to your systems, hides from detection by learning, and finds valuable targets automatically.
Adaptive malware shows this in action. These programs constantly change their code. They keep working while looking different to security tools. Protection systems struggle to catch these moving targets, and the advantage shifts to the attackers.
Ransomware groups now use AI capabilities. They automate finding weaknesses, set ransom amounts based on what victims can pay, and create convincing messages. The attacks become more efficient and effective.
Automated Attack Optimization
AI lets attacks improve automatically. Systems test different approaches quickly. They learn which techniques work best against specific targets. They adjust strategies based on success rates. The attacks grow smarter through experience.
A manufacturing company saw this evolution firsthand. Their security team noticed increasingly focused attempts. The attacks tested different systems methodically. Each wave learned from previous failures. The pattern suggested AI-driven coordination behind the scenes.
These capabilities once belonged only to nation-states. Now, criminal groups use them too. The spread of AI tools expands the danger. More attackers gain access to advanced capabilities.
Privacy Risks and AI Data Breaches
AI systems process huge amounts of sensitive information, which creates new privacy dangers. Training data might leak personal details, model outputs could reveal confidential information, and the connections between seemingly anonymous data points might identify individuals.
A healthcare analytics firm faced this exact problem. Their AI system started revealing patient information patterns. The details appeared in seemingly unrelated reports. The investigation showed that the model had memorized specific cases. Patient privacy was compromised without any traditional data breach.
These risks grow as models become more powerful. Large language models remember portions of their training data, and image generation systems keep elements of reference materials. The line between training and privacy breach becomes increasingly blurry.
How to Protect Yourself from AI Risks
The threats sound scary, but practical defenses exist. Companies can protect themselves through smart strategies, and individuals can reduce their risks. The key lies in updated security approaches.
My experience helping dozens of organizations suggests five critical areas. Each needs attention in the AI security landscape. Together, they provide real protection against emerging threats.
Develop an AI Security Strategy and Governance Framework
Every organization requires a complete AI security strategy. Organizations must establish clear governance procedures that represent the beginning of their AI security approach. Which authority leads the security protection of AI? What policies guide implementation? What are the procedures for identifying risks plus their remediation methods? These questions need specific answers.
Regular security checks become essential. Standard evaluation processes do not detect unique vulnerabilities in AI systems. Testing protocols need to focus on threats unique to these systems. Experts outside the organization can supply critical perspectives about system performance.
Documentation matters more than ever. AI systems transform because of usage and behavioral modifications that occur during system operation. Comprehensive documentation brings achievable oversight capabilities that also enable auditing procedures and satisfy regulatory standards.
Invest in AI Security Talent and Training
The insufficient number of available AI security experts is a major organizational challenge. Organizations encounter difficulties when they search for qualified personnel. The knowledge gap creates weakness. Active talent development is an effective solution to overcome this problem.
Security teams that receive training can help organizations meet their urgent security requirements. These professionals need to be taught AIT basics. Security training programs exist for AI specialists to enroll in. The union of these two elements results in enhanced protective measures.
Outside partnerships fill capability gaps. Strategic consulting companies provide focused expertise to clients. Academic partnerships bring cutting-edge knowledge. Industry groups share threat information. No organization can manage alone.
Improve Privacy and Data Protection Systems
Data protection needs renewed attention. Implementing AI technologies introduces new privacy concerns that existing control methods tend to fail to address. New approaches need to define the information access processes and use methods of AI systems.
Access controls need careful design. What data can each system access? How is usage tracked? What approval processes govern new connections? Clear boundaries reduce risk exposure.
Data minimization principles have become more important. Systems should access only necessary information. Training data requires careful screening. Personal details should be anonymized when possible. Less accessible data means fewer vulnerabilities.
Invest in AI Ethical and Security Training
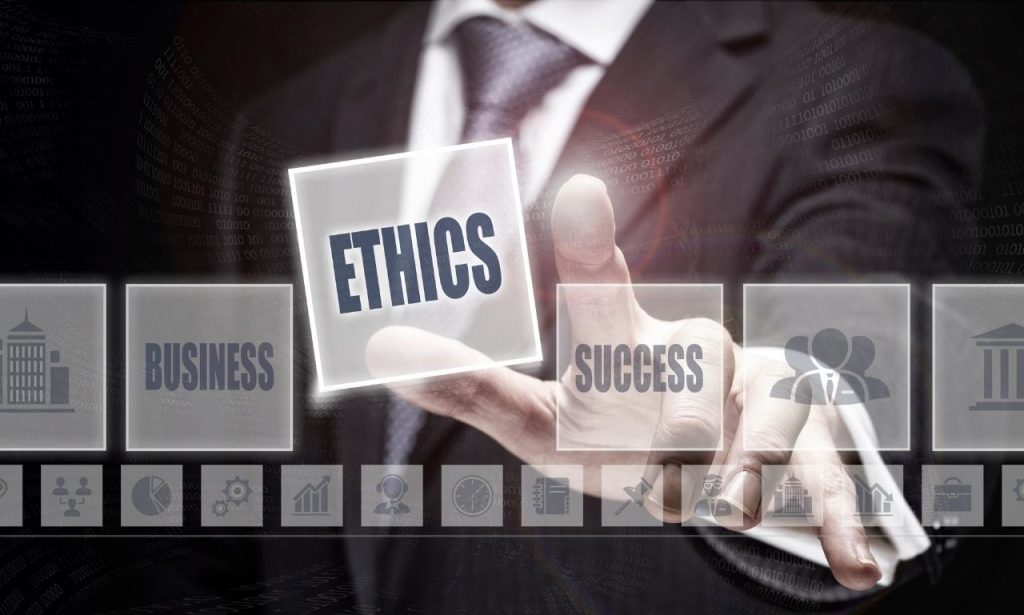
Human judgment remains our strongest defense. Well-trained employees spot suspicious activities, question unusual requests, and follow verification steps. Investment in training pays major dividends.
Security awareness programs need AI-specific components. Employees should understand new threat types, recognize AI-generated communications, and know how to report suspicious activities.
Ethical frameworks guide responsible AI development, help identify potential weaknesses, encourage security-focused design, and support organizational values while reducing risks.
Conclusion
AI-developed security threats have introduced completely new challenges to the field of cybersecurity. The new technologies enable attackers to build innovative methods for their attacks. They enhance existing threats. These systems achieve operation at levels unmatched by previous systems and complexity at never-before-seen thresholds.
But the situation isn’t hopeless. Innovative strategies reduce these risks. Updated security practices deliver actual protection against threats. The combination of human expertise with technical security measures generates adequate defensive measures.
Recognition, combined with preparation, is the key factor. Organizations must recognize the present situation, which requires them to adopt modern security strategies and invest in technology and people.
The coming years will bring both new threats and better defenses. The security community continues developing countermeasures. Regulations evolve to address emerging risks. Awareness grows among potential targets.
Your security depends on staying informed and prepared. Use the recommendations in this article. Work with security professionals who understand AI threats—question unusual digital communications. The threats are real, but so are the solutions.
Also Read: How AI Has Revolutionized Financial Forecasting
FAQs
AI attacks adapt to defenses, operate at a massive scale, and create more convincing scams. They learn from successes and failures while requiring less human intervention.
Look for unusual urgency, verification avoidance, slight tone inconsistencies, and requests that bypass normal procedures. Always verify unusual requests through separate channels.
Yes. AI tools make sophisticated attacks accessible to more threat actors. Small businesses often have valuable data with fewer security resources.
Absolutely. AI improves threat detection, analyzes patterns, automates responses, and enhances security monitoring. The technology works for both offense and defense.